How do you measure how engaged the audience is with a piece of content? Why did the audience member leave before it finished? Jonathan Carlton, an iCase PhD student with The University of Manchester was exploring these types of questions at ±«Óãtv Research & Development, within the context of interactive media experiences, for example, Origami Frog Make-Along and Instagramification. In this post he investigates whether these questions can be answered using an audiences’ clicks with interactive media.
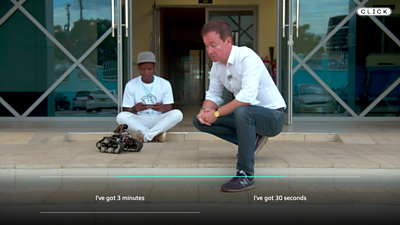
We need to understand how effective the experiences we create are, so that we can build on our successes and learn from our failures. In my research, I have been investigating whether or not the interaction data (buttons pressed and the choices made) can give us clues about how engaging people find the content, or how likely they are to abandon it. Using this kind of data has the benefit that we can capture it from the whole audience, at scale, and unobtrusively - an advantage over traditional methods, such as lab-based studies and focus groups.
In this post I’ll focus on two studies that we carried out on these types of media, one to investigate the engagement of users, and another to develop an understanding of content abandonment and its relationship to engagement. By gathering an understanding of engagement and abandonment, it provides a method for newly produced interactive experiences to be evaluated.
For both studies, we anonymously collected the clicks of users from the national release of the one-thousandth special edition of Click, a long-running ±«Óãtv technology show (shown above). This edition was an interactive branching narrative where the audience could make choices (prompted by the on-screen host; shown above) to determine their path through the story based on their interests, and it focused on technology use in Malawi and autonomous vehicles. The experience provided next and back buttons, enabling the audience to move to the next or previous segment of content (called narrative elements). Within a narrative element, the usual video controls could be used to move the video play-head or to replay the narrative element. These interactions with the experience, along with the choices made, were captured and form the datasets used in the two studies.
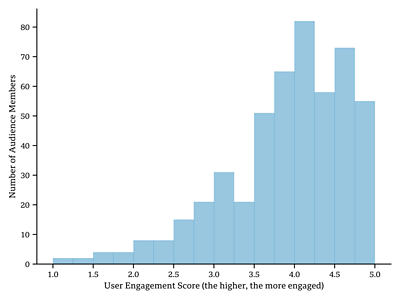
User Engagement
Knowing how engaged the audience members were with an experience is a key metric to evaluate success. User engagement is complex to measure and there are established approaches in other domains, but how these translate to interactive media experiences is unknown. To address this, we attached an established survey () to Click to quantify engagement and investigated whether there were links between the clicks of users and engagement levels indicative of user behaviours.
We found that the audience were engaged with Click (see above), with a positive skew towards the higher end of the engagement score scale. Through training a machine learning model to predict high/low engagement and investigating what metrics the model relies on to make predictions, we found that low engagement audiences tended to skip through the content and interacted more regularly (a skipping-type behaviour). In contrast, highly engaged audience members typically interacted less. As the content is similar to a traditional TV show (even if it is more interactive), we believe this shows a focus on the content and audience members only interacting when necessary (a consumption-type behaviour). On the other hand, skipping through the content indicates lower engagement and could be analogous to fast-forwarding a video.
Content Abandonment
The analysis in the previous study focused on the audience members that completed the engagement survey, here we expanded the analysis to the wider dataset and investigated whether abandonment could be predicted from the user interactions. Abandonment is where audience members drop out of the content before reaching its end and is typically a signal of a negative experience. Focusing on the two main sub-stories in Click and to quantify abandonment, we used the graph-based nature of the story and counted how far away from the end each user (that abandoned) was when they left - higher values indicate being further from the end.
Like the previous study, we trained a machine learning model to accurately predict abandonment. By investigating what metrics the model relied on, we found that the more someone’s browser visibility changes - where the web browser tab becomes visible or hidden - increased the likelihood of the model predicting abandonment. Additionally, the more someone moved backwards and forwards between narrative elements also increased the likelihood of abandonment. We believe this type of behaviour might be seen when people are exploring the content, to see what is available or how it works, and these types of users are less likely to watch to the end than people who just sit down and watch.
We also investigated whether there was a relationship between engagement and abandonment, finding that audience members that reported a high level of engagement were consistently predicted to be less likely to abandon. The inverse was true for audiences that reported a low level of engagement, and potentially establishes a link between engagement and abandonment.
Conclusion
Detecting the types of behaviours found in the analysis could provide content creators with a detailed picture of the success of their experience and the means to retrospectively understand what types of story experiences work better. For example, identifying a loss of engagement during the experience and detecting skipping could provide the opportunity to modify an experience on-the-fly to provide a shorter, summarised version of the narrative. Similarly, identifying these behaviours in real-time could enable the capture of additional data to provide a more nuanced understanding of the rationale behind abandonment.